Leaders in Lending | Ep. 46
The Steady Adoption of AI & Machine Learning in Credit & Banking
In this episode of Leaders in Lending, Tom Faughnan, EVP & Director of Consumer Lending at Associated Bank discusses the potential of AI and machine learning in banking, why some financial institutions have been slow to adopt and the repercussions of lagging behind.
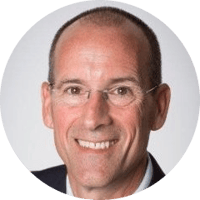
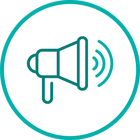
GUEST SPEAKER
Tom Faughnan
Key Topics Covered
- The opportunities and hurdles of AI & ML in credit decisioning
- How to find the right fintech partner in the space
- Aligning internal parties across business lines during the vendor management process
- Navigating the mortgage space as rates rise
"We are farther ahead in creating a machine learning environment, particularly when it comes to credit decisioning.”
“Models open up greater opportunities to provide credit to traditionally unbanked, unlent markets.”
“You've got to find a way not only to use technology effectively to create a great customer experience, but to be able to support when a customer says, I want to talk to somebody.”
EPISODE RECAP & SUMMARY
When lenders apply artificial Intelligence (AI) and machine learning (ML) to their lending processes, it leads to major results.
With the ability to connect more data points, AI can improve underwriting systems, expand an institution’s borrower base and grant more consumers financial aid.
It’s no question that AI has multiple benefits in the lending space, so why are banks hesitant to adopt?
In this episode of Leaders in Lending, Tom Faughnan, EVP & Director of Consumer Lending at Associated Bank discusses the potential of AI and machine learning in banking, why some financial institutions have been slow to adopt and the repercussions of lagging behind.
We discuss:
- The opportunities and hurdles of AI & ML in credit decisioning
- How to find the right fintech partner in the space
- Aligning internal parties across business lines during the vendor management process
- Navigating the mortgage space as rates rise
Regulation and AI
The reason bigger institutions have been slow to adopt AI and ML is due to a hesitancy from regulators.
Uncertainty around the outcomes of these models is the main inhibitor, so in order to assuage those worries, it’s important to give it time to prove that these technologies are reliable and are helping bring more customers to the base.
Additionally, these models are designed to leverage non-traditional data that larger institutions aren’t used to relying on for credit decisioning.
Underbanked markets & fair lending
The beauty of AI and ML in the lending space is that it also opens up greater opportunities to historically underserved markets.
Traditional underwriting processes exclude a large pool of consumers that could be trusted to finance a loan.
While fair lending laws are set up to protect marginalized communities, it isn’t enough to distribute more help.
Operationalizing any model that measures credit with more precision and provides more people with more aid, is a major incentive.
Financial institutions can take comfort in the idea that by employing these technologies, they help their communities build households and families.
Finding the right fintech partner
While it’s alluring to build an AI that’s capable of reimagining traditional processes, it’s not always simple knowing where to start.
The expertise needed to design that type of model can only be found in partnership with a fintech company.
However, finding the right fintech provider is no easy task.
Since most fintechs are at an early stage, they’re typically still unprofitable and VC-funded, so doing the research and determining which ones will evolve into a profitable business is critical.
Traditional vendor management systems aren’t designed to invest in organizations that look unprofitable and thus, may or may not succeed in the future.
Vendor management process
So how do banks find fintech partnerships that are right for them?
Faughnan suggests that the first step is to really, “understand the landscape, the true benefits the fintech will bring to your organization, and then you've got to be able to say, ‘what is your path to profitability?’”
Having a complete vision of their business plan, whether or not their investors are reliable, and potential exit strategies are more steps to consider when measuring the risk of an investment.
From there, it’s important to get all vendors—credit risk, IT, business, etc.—to have an open, collaborative conversation and weigh in on all the potential benefits and risks of investment.
According to Faughnan, “you want to make sure you vet all the risks, you understand the risk, you've mitigated the risk, and then you stand back and go okay, is my residual risk and the reward adequate?”
Chatbots
While the financial world is focused on a migration to digital banking and improving that process, sometimes basic consumer-technology interactions fall by the wayside.
“At the end of the day, the person on the other end of the transaction is a person,” said Faughnan.
Not only must financial institutions find a way to integrate new technology effectively and create a reliable customer experience, but they need to be able to support them when they need guidance from a human being.
Chatbots are a form of AI and machine learning that can be effective, but have a long way to go before they could be considered useful.
In a digital world where services are constantly evolving, ensuring that customers have easy access to help is the best way to serve them.
Stay tuned for new episodes every week on the Leaders in Lending Podcast.