Rising interest rates
The State of Lending:
Q3 2022
Long-term risks and opportunities
Introduction
The uncertainty in our current economic environment has caused some lenders to tighten their approach to credit and pull back from some lending activities. However, I believe that this is a moment when AI can help lenders best serve their communities while properly managing the risk and returns in their portfolios - resulting in higher approval rates and lower cost of credit.
Rather than relying on traditional qualifiers alone, such as credit score and debt-to-income ratio, lenders can assess these alongside thousands of other variables to generate much more accurate predictions about a borrower’s likelihood to repay a loan. Furthermore, lenders can create seamless, embedded experiences that create stickier customer relationships while also attracting new customers and members.
During uncertain times, lenders can make short-term decisions that come at the cost of long-term strategic priorities. Given the cyclical nature of the economy, investing in technology during turbulent times is the best way to take advantage of opportunity during better times. Rather than inaction or scaling back, banks and credit unions that double down on digital transformation and disruptive technologies like AI and machine learning can not only weather challenging times, but continue to grow.
Jeff Keltner
Senior Vice President
of Business Development
Upstart
“Software is eating the world,
but AI is going to eat software.” 1
Jensen Huang
CEO, Nvidia
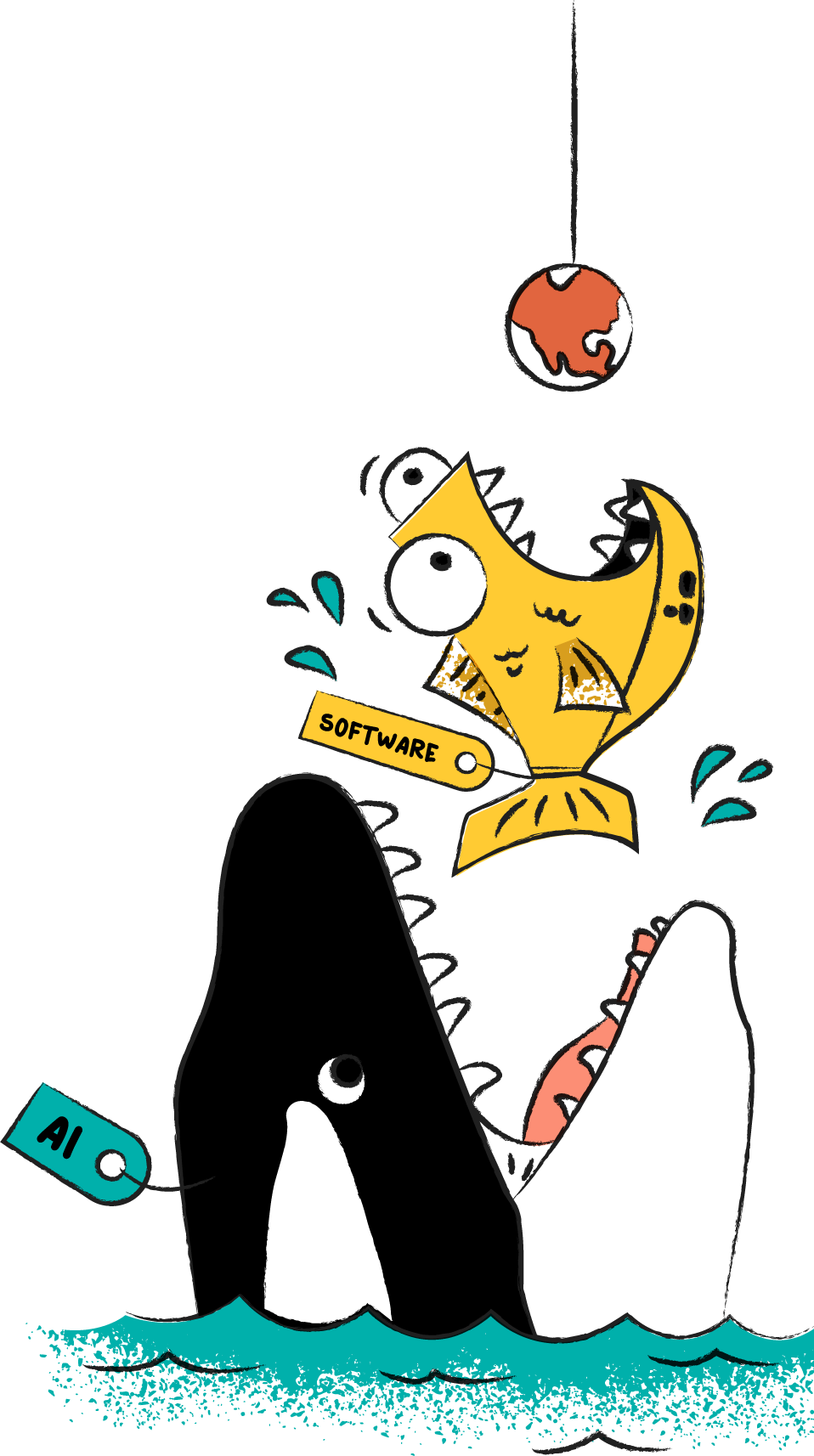
Short-term and long-term risks
Recent economic headwinds have made lenders especially nervous to adopt alternative lending methods.
A combination of the following circumstances have left the credit environment more conservative than 2021:
Inflation
The war in Ukraine
The end to the largest stimulus in recent history
As Americans have increased their spending in 2022, their desire for consumer credit is now returning, also fueled by increasing prices. The Federal Reserve reported the lowest savings levels and a record 537 million credit card accounts open in Q1 2022.2
As mortgage purchases and refinances begin to dry up as interest rates rise and borrowers seek quick access to capital for things like home improvements and debt consolidation, lenders who want to safely grow will seek out new areas of opportunity to meet growing customer and member needs.
75% of bank and
credit union
executives are
increasing their innovation
spending in the
next 12 months.
For example, personal loans offer faster access to capital than home equity lines and are the fastest growing category of consumer credit. Lenders are starting to raise their return targets and therefore increasing prices. Consumer demand remains strong, and tech-savvy banks and credit unions that develop fintech relationships in this space are continuing to see consumer and loan growth. In fact, 75 percent of bank and credit union executives are increasing their innovation spending in the next 12 months.3
Another example is within auto lending. Specifically, auto refinance loans represent a largely untapped opportunity with tremendous opportunity for growth; in fact, only 12 percent of car buyers have ever refinanced an auto loan4 while the refinance share of mortgages is about 40 percent.5 This showcases the massive untapped opportunity in auto refinance. While interest rates have risen, there is still a significant opportunity for banks and credit unions to save borrowers money by helping refinance poorly-priced new and used car auto loans obtained from other channels.
As lenders look to increase their loan market share in these products, they must balance this growth appropriately against ongoing risk of inflation and recession, especially now that excess savings from stimulus has dried up. Therefore, lenders must not only offer a best-in-class digital experience, but augment their processes with AI and machine learning in order to better assess and identify creditworthy borrowers while mitigating risk.
Leaders at financial institutions who make strategic investments in digital and AI and machine learning during this period of economic downturn will emerge in a stronger position when markets stabilize. As proof, 46 percent of 80 surveyed credit union leaders are already using or plan to use AI in the next 12 months.6 Additionally, 75 percent of banks over $100 billion in assets and 46 percent of banks under $100 billion in assets are currently implementing AI.7
46% of credit union
leaders are already using
or plan to use AI in the
next 12 months, and 46%
of banks under $100
billion are currently
implementing AI.
Winning the
modern consumer
There are three key trends shaping consumer experiences and the market in which lenders compete in today:
1) Increased competition
Gone are the days when a consumer would first walk into the closest branch and consult with a lender face-to-face. Today’s consumers prefer to rate shop, and online lenders make it easier than ever to compare rates instantaneously. Moreover, many of these online lenders have a national footprint, so financial institutions are not just competing locally, but on a national scale. In order to win these consumers, lenders can:
- Offer the best rates - As rate shopping is an increasingly common reality, lenders must leverage better risk-based pricing models to help them win borrowers more frequently.
- Provide better experiences - In addition to being rate sensitive, consumers are also effort sensitive. In fact, the best borrowers tend to be the most sensitive to the lending experience, making this important for lenders to get right.
- Leverage better distribution - Borrowers increasingly want to borrow where they’re transacting, putting increased pressure on lenders to make the right offer to the right consumers at the right time. Lenders can leverage data for better customer and member targeting and partner with fintechs that specialize in this area.
2) Seamless experiences
With the standard being set by companies like Amazon, Netflix and Uber, today’s borrowers expect truly seamless, digital experiences. However, lenders often make the following mistakes:
- Too much focus on the member-facing experience - Many banks and credit unions are hyper-focused on polishing the front-end consumer experience while the underlying back-end processes remain manual for both the bank or credit union and consumer. For example, while applications may be online, consumers still have to bring it into the branch or speak with a lender representative in order to move forward.
- Weak infrastructure - Without investment in the back-end data, analytics and interconnectivity, lenders cannot deliver on the digital experiences their front-ends are offering.
- Can only serve existing members - Some lenders only offer a digital experience to their current customers and members when this needs to be consistent across both existing and new borrowers. Despite the overarching need to get this digital experience right for all, 55 percent of leaders say their organization’s strategy is often or always outpaced by the demands of the business.8 To prevent this gap from widening even further, lenders need to take a proactive approach to digital transformation and technology investment that attracts new customers and members and serves existing consumers.
3) Embedded lending
Consumers want to borrow where they’re transacting and understand when they are borrowing and under what terms. As consumer purchasing shifts to digital channels and spending-related purchasing, there has also been a shift from credit cards to buy-now-pay-later transactions. As a result, lenders need to evaluate their buy-now-pay-later strategies, including potential partnership opportunities.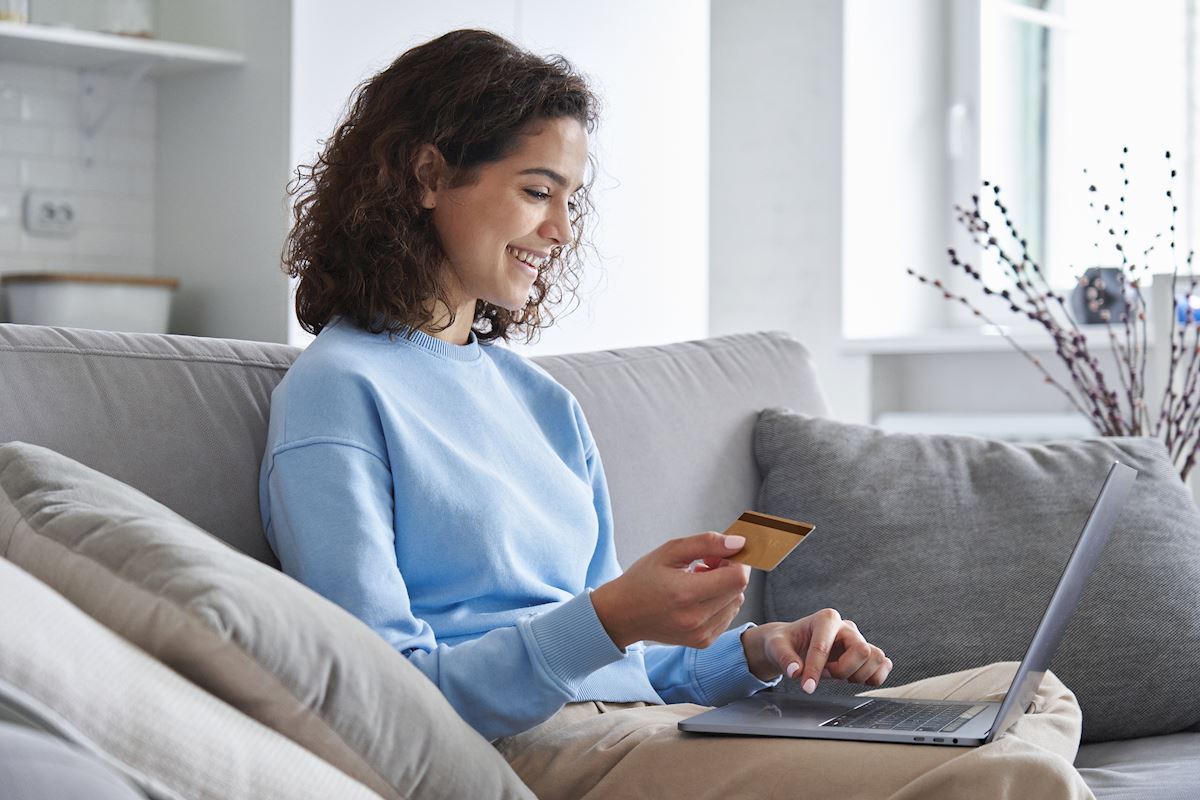
Leveraging AI in tough
times to navigate risk
While lenders have traditionally reacted to market turbulence by tightening their credit standards and increasing their interest rates, AI and machine learning models present the opportunity for a more targeted approach to assess consumer creditworthiness to lend safely while enabling lenders to contribute to a more seamless and inclusive credit system.
1) Real-time models that consider macroeconomic conditions - By leveraging a model that considers macroeconomic factors, such as unemployment rates, lenders gain a fuller understanding of where there is increased risk. AI lending platform providers enable lenders to continue to meet their loan volume and loss targets since the models employ stress testing using historical data and adjust according to macroeconomic conditions – the model updates in real-time rather than remaining stagnant.
2) Full control over risk and returns - With a targeted risk assessment that changes based on macroeconomic conditions, banks and credit unions can target borrowers that fall within their desired risk tolerance and adjust accordingly as their goals and risk appetite change. Similarly, given the level of risk taken on, lenders can turn the dial on their needed returns. Even amidst the current macroeconomic environment, lenders on Upstart’s platform have continued to meet their desired volume, return and loss rate targets.
Upstart’s model
approves 43% more
Black borrowers
and 46% more
Hispanic borrowers
than traditional
scoring models.
3) Create smarter and more inclusive lending decisions - Many lenders worry that lending more inclusively is synonymous with taking on higher amounts of risk, yet AI and machine learning models employ thousands of data points to improve the accuracy of measuring credit risk. Upstart rigorously tests its models in production for bias and accuracy and has a strong model governance program that focuses on model explainability.
It is also important to employ rigorous testing on every loan and application for fairness. By leveraging AI, lenders can improve access to credit and contribute to a fairer and more equitable financial system, even in challenging times. In fact, Upstart’s model approves 43 percent more Black borrowers and about 46 percent more Hispanic borrowers than traditional credit-score only models.9 Additionally, 30 percent of borrowers who received a loan through Upstart with a credit score of less than 680 would have been rejected by traditional models.10 11
4) Unlock seamless credit experiences - Ultimately, AI can improve all aspects of the lending process, from application, through credit decisioning, fraud protection and verification and servicing.
Application
- Firstly, lenders can reach and attract the right consumer at a lower cost. By assessing both transaction and credit history, machine learning models can predict the optimal product and time to offer that product to a borrower to ensure the highest
Credit decisions
- When it comes to credit decisioning, lenders can price risk accurately with thousands of data points on borrowers, detailing who paid back and when, as well as predict if and which month an applicant may fail to make a payment. When compared to pre-existing credit policies, Upstart’s AI models resulted in a 173 percent increase in approvals at the same loss rate.12
Fraud protection and verification
- Applied to fraud protection and verification, AI can decrease manual processing and increase approvals. In fact, more than 70 percent of Upstart’s loans are approved with no documentation or phone calls necessary.13 Additionally, Upstart’s fraud detection has limited fraud to <0.3 percent.14
Servicing
- Finally, when it comes to servicing, lenders can predict who will become delinquent and even tailor messaging most effective at limiting borrowers at risk of defaulting on their credit obligation. By understanding who is more likely and less likely to default using AI models, lenders can differentiate their servicing messaging and touchpoints, allowing them to prioritize borrowers that are potentially at higher risk of defaulting versus those who are lower risk.
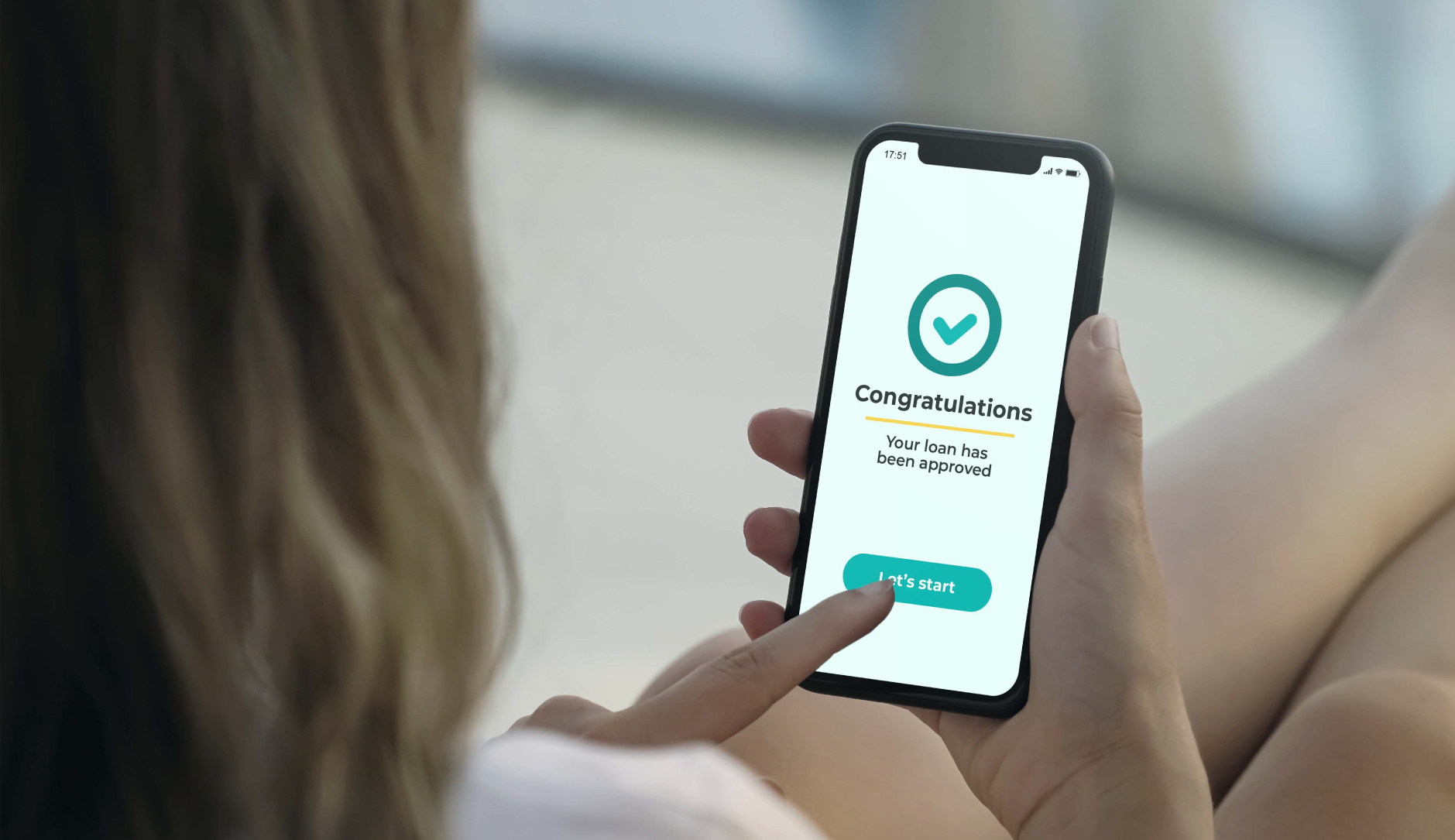
Conclusion
With rising interest rates, high inflation and uncertainties about economic recession on the horizon, banks and credit unions need to be doubling down on innovations, like digital transformation and applying AI and machine learning to the lending process. Though some institutions are in the midst of business model changes and cost-cutting, banks and credit unions need to be strategic about which areas of investment will deliver the greatest long-term value.
By leveraging an AI lending platform, lenders are empowered to make their own decisions and adjustments about risk tolerance and reward in the current environment. Though these providers give banks and credit unions the information about the general market, average return yields and more, it is ultimately up to the individual lender to make their own decision based on their business goals and risk tolerance at any point in time.
A lending approach that combines a seamless borrower experience with machine learning models that factor in macro-driven adjustments will enable banks and credit unions to best serve their customers and members and stay profitable during turbulent times.
1 MIT Technology Review. “Nvidia CEO: Software Is Eating the World, but AI Is Going To Eat Software.” May 12, 2017.
2 Bloomberg. “Americans Put More on Credit Cards as Inflation Boosts Costs, Fed Data Show.” May 10, 2022.
3 Financial Brand. “Banks Boost Innovation Budgets and Shift Focus From CX to Operations.”June 10, 2022.
4 Wolters Kluwer. “A closer look at the auto refinance market for lenders.” June 3, 2019.
5 CNBC. “Mortgage Refinance Demand Plunges 60%, as rates hit their highest level since 2018.” March 30, 2022.
6 NAFCU. “State of Lending: Long-Term Risks and Opportunities” webinar survey. June 11, 2022.
7 Business Insider. “The Impact of Artificial Intelligence in the Banking Sector & How AI is Being Used in 2022.” Feb 2, 2022.
8 Workday. “Closing the Acceleration Gap: Toward Sustainable Digital Transformation.” 2022.
9 Harvard Business School. “Invisible Primes: Fintech Lending with Alternative Data.”October 2021.
10 Ibid.
11 As of December 31, 2021, and based on a comparison between the Upstart model and a traditional credit-score only model. Upstart does not collect demographic data on borrowers. Upstart uses standard industry methodology to estimate borrower demographic status to conduct access-to-credit analysis comparing Upstart to traditional credit model outcomes.
12 In an internal study, Upstart replicated three bank models using their respective underwriting policies and evaluated their hypothetical loss rates and approval rates using Upstart’s applicant base in late 2017. Such result represents the average rate of improvement exhibited by Upstart’s platform against each of the three respective bank models.
13 As of 3/31/2022. Fully automated loans are defined as loans originated end-to-end (from initial application to final funding) with no human involvement.
14 Based on an internal Upstart data analysis of personal loans first payment defaults as of 9/30/2020.