Leaders in Lending | Ep. 59
AI Lending 201: The Evolution of AI and Machine Learning at Upstart
Jeff Keltner, Senior Vice President of Business Development at Upstart, discusses the many lessons learned from Upstart's journey applying machine learning techniques to the lending environment.
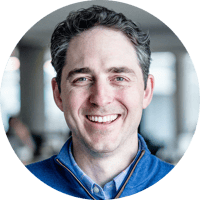
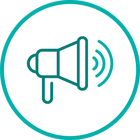
GUEST SPEAKER
Jeff Keltner
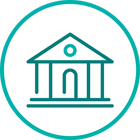
ABOUT
Upstart
Key Topics Covered
- Challenges to overcome at the beginning stage of the ML journey
- Why feature engineering and first-party data build on each other
- The evolution of Upstart’s underwriting model
- Shifting from manual to automatic identity verification
- How to attain more interesting predictions and apply them in the credit industry
- Mitigating risk with creativity
"Evolution is a core part of how machine learning in the real world works. You have to start simple with the capabilities and data that you have and build and mature over time."
"Think really long and hard about how and where to apply machine learning and how well-suited the problems and data you have are to the application of these technologies."
“Machine learning is easy to do at a certain level, but doing it at the highest levels takes time, effort and dedication.”
EPISODE RECAP & SUMMARY
Upstart has learned many lessons from its journey applying machine learning (ML) techniques to the lending process.
Here are the major takeaways from Upstart’s ML journey, including how the company overcame initial challenges, evolved over time and effectively mitigated risk.
Leaders in Lending host Jeff Keltner, Senior Vice President of Business Development at Upstart, discusses:
- Challenges to overcome at the beginning stage of the ML journey
- Why feature engineering and first-party data build on each other
- The evolution of Upstart’s underwriting model
- Shifting from manual to automatic identity verification
- How to attain more interesting predictions and apply them in the credit industry
- Mitigating risk with creativity
Early challenges in the ML journey
Upstart came into existence based on one core belief: AI can fundamentally improve credit outcomes, and by doing so, widen the pool of creditworthy Americans to include historically underserved populations.
Doing so would require moving beyond traditional approaches of assessing creditworthiness—and ML techniques represented the path forward.
In the early days, the company encountered some common challenges among companies setting off into the ML space.
The kickstart problem
Ideally, first-party data is ideal for training models, but in the case of Upstart, first-party data required making a loan. As a new company in the space, Upstart hadn’t.
So, Upstart's first models incorporated only a small number of variables taken mostly from third-party data. Upstart used data from outside lenders, like credit bureau retros and publicly available data from lending platforms.
“We had a beginning stage where we didn’t have enough data to use a lot of variables, not enough data to use the most sophisticated techniques, and not enough data to do really complicated predictions”, Jeff says. “Yet, we could build a model and that model showed really tremendous uplift.”
Limiting risk
Another challenge Upstart faced was finding creative ways to limit risk early on. Because Upstart was using a model that was new and untested, they leveraged cross- validation and accuracy metrics to limit the chances of model inaccuracy.
They leveraged other tactics as well, including implementing harder requirements into the credit policy.
“We started off with relatively high traditional credit score requirements and relatively low debt-to-income thresholds,” Jeff says. “But that gave our partners, on the lending side and on the investing side, some confidence that we had controls around the risk of the model being accurate.”
In time, Upstart gained more confidence in the accuracy of the model, which enabled them to gradually loosen those requirements.
More first-party data, more feature engineering
As Upstart continued to use the model, they obtained more first-party data. With greater levels of first-party data, they started to use more sophisticated techniques, like gradient boosting, and find higher-order interaction effects.
Plus, with more data, Upstart could continue finding new ways to combine their variables—a practice known as feature engineering.
“Think of feature engineering as a shortcut for the model to say, we know that this combination of variables is really predictive,” Jeff says. “So, we’re going to add it as a new variable to the model.”
In essence, it becomes a flywheel. As more variables are added to the model, the model becomes more accurate, resulting in more first-party data which contributes to more feature engineering.
Evolution of Upstart’s underwriting model
Throughout the ML journey, incremental improvements are achieved, but every now and then, there are moments that represent massive leaps forward in effectiveness. Upstart had two critical moments that fit into that category.
The first was the switch from third-party data to first-party data.
“When we switched, there was a large increase in accuracy and a moment where we really felt like, in some way, we were starting to control our own destiny a little more, because you have your own data training the model,” Jeff says.
The second was the shift to more interesting predictions.
Because of the growing complexity of the model and the steady increases in data, Upstart could move beyond simple lending predictions, such as whether a person will pay back the loan or not. Now, they could dig deeper into the data to make more complicated predictions.
“We moved from that binary prediction, to a probability of default, to understanding the real components of the economics to a lender of the loan—not only will this person default or not, but at what time,” Jeff says.
Looking at that timing curve was a game-changer for the company. It enabled them to make better risk predictions and better decisions.
“We see so much opportunity left between feature engineering, new data sources, maybe new credit bureau data sources with alternative points of data, different things we can do to improve the predictive power of that model and improve our ability to help our partners serve consumers and to make sure they're earning the right economic rewards for the risk that they're taking,” Jeff says.
Automatic identity verification
Another area where Upstart applied ML techniques is identity verification.
In the early days, the company performed manual verifications. It was another way to limit the risk and verify the accuracy of the model. Around 2016, they began to imagine a world in which fully automated approval existed and decided to give it a try.
They launched a pilot with a small percent of borrowers and only small dollar loans and received some interesting results:
- There was a 2-3x increase in conversions.
- They also learned that good borrowers not only want to see a good rate; they also don’t want to put in a lot of effort.
Given these new findings, Upstart started to look at this idea as an ML problem.
“We've pointed the the engine of machine learning, if you will, towards how do we identify those applicants, where the automated data sources that don't introduce user friction, actually provide us sufficient data to do all the verification work,” Jeff says.
At its core, it’s a harder problem to solve than underwriting because the data is less clean and the outcomes are less clear. But Upstart has seen great results from applying ML to it.
3 big takeaways
- ML is not a magic wand - There’s a difference between doing ML and doing ML well. It takes work, iteration and dedication to get it right. It’s not one and done; it’s a process.
- Think hard about how and where to apply ML - Some problems aren’t well-suited to ML. Think about where you can get the best results and the best return on investment in the early days of the journey.
- Be creative in how you control risk - Institutions need to figure out how to fit ML into their overall process and find the right levers to control and manage risk so that they can continue to learn and iterate into more expansive use cases.
Stay tuned for new episodes every week on the Leaders in Lending Podcast.